Explore
CATEGORIES
POPULAR SOURCES
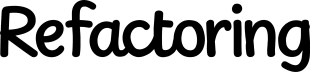
Refactoring
Luca
Refactoring is like a personal coach that helps you write great software and work well with humans — only for real cheap! It is read every week by 140,000+ engineers and managers
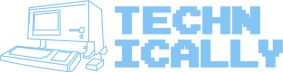
Technically
Justin
Our lives are dominated by software, but we don’t understand it very well

The System Design Newsletter
Neo Kim
The System Design Newsletter
Daily Dose of Data Science
Many Authors
Daily column with insights, observations, tutorials, and best practices on data science.

Architecture Notes
Mahdi Yusuf
System Design & Software Development
Pro Tip
Create a free account to save articles, follow your favorite sources, and get personalized recommendations.
Create Free AccountBrowse Categories
Latest Technical Insights
Stay up to date with the latest developments in software engineering, system design, AI, and more.
How Load Balancing Algorithms Really Work ⭐
Load balancing algorithms distribute network traffic across multiple servers to optimize resource use, maximize throughput, and ensure reliability. The article explores static (e.g., Round Robin, Weighted Round Robin) and dynamic (e.g., Least Connections, Least Response Time) algorithms, their use cases, and trade-offs. Key considerations include performance, scalability, and fault tolerance in distributed systems. --- ### Core Technical Concepts/Technologies - **Load Balancing**: Distributing traffic across servers to improve efficiency. - **Static Algorithms**: Fixed rules (e.g., Round Robin, IP Hash). - **Dynamic Algorithms**: Adapt based on real-time metrics (e.g., Least Connections). - **Health Checks**: Monitoring server availability. - **Session Persistence**: Maintaining user sessions on the same server. --- ### Main Points - **Static Algorithms**: - **Round Robin**: Cycles through servers sequentially; simple but ignores server load. - **Weighted Round Robin**: Assigns traffic based on server capacity (weights). - **IP Hash**: Uses client IP to map to a server, ensuring session persistence. - **Dynamic Algorithms**: - **Least Connections**: Routes to the server with the fewest active connections. - **Least Response Time**: Combines connection count and latency for optimal routing. - **Resource-Based**: Consumes server metrics (CPU/RAM) for decisions. - **Implementation**: - Health checks prevent routing to failed servers. - Session persistence is critical for stateful applications (e.g., e-commerce carts). - **Trade-offs**: - Static: Low overhead but less adaptive. - Dynamic: Higher performance but complex to implement. --- ### Technical Specifications/Examples - **Round Robin Code Snippet** (pseudo-code): ```python servers = ["s1", "s2", "s3"] current = 0 def next_server(): global current server = servers[current % len(servers)] current += 1 return server ``` - **Weighted Round Robin**: Assign weights like `{"s1": 3, "s2": 1}` for 3:1 traffic distribution. --- ### Key Takeaways 1. **Choose static algorithms** for simplicity and predictable workloads (e.g., Round Robin). 2. **Dynamic algorithms** (e.g., Least Connections) excel in variable traffic environments. 3. **Session persistence** is vital for stateful applications; IP Hash or cookies can achieve this. 4. **Monitor server health** to avoid routing traffic to failed nodes. 5. **Weigh trade-offs**: Dynamic algorithms offer better performance but require more resources. --- ### Limitations/Further Exploration - **Static algorithms** may overload servers if weights are misconfigured. - **Dynamic algorithms** introduce latency due to real-time metrics collection. - **Hybrid approaches** (e.g., combining Least Connections with weights) could be explored.
How Google Measures and Manages Tech Debt
Google employs a structured framework called DORA (DevOps Research and Assessment) to measure and manage technical debt, focusing on four key metrics: deployment frequency, lead time for changes, change failure rate, and time to restore service. These metrics help teams balance innovation with stability while systematically addressing technical debt through prioritization and incremental improvements. The approach emphasizes data-driven decision-making and cultural shifts toward sustainable engineering practices. ### Core Technical Concepts/Technologies - **DORA Metrics**: Deployment frequency, lead time for changes, change failure rate, time to restore service - **Technical Debt Management**: Quantification, prioritization, and incremental reduction - **Engineering Productivity Metrics**: Code quality, system reliability, and team velocity - **Data-Driven Decision Making**: Metrics aggregation and visualization (e.g., dashboards) ### Main Points - **DORA Metrics Framework**: - Measures software delivery performance using four core indicators. - High-performing teams deploy frequently, recover quickly, and maintain low failure rates. - **Technical Debt Management**: - Quantified using metrics like code churn, defect rates, and incident frequency. - Prioritized based on impact vs. effort, addressed incrementally (e.g., "20% time" for debt reduction). - **Engineering Culture**: - Encourages blameless postmortems and shared ownership of system health. - Tools like Code Health dashboards track debt trends and team progress. - **Implementation**: - Integrates metrics into CI/CD pipelines (e.g., monitoring lead time via deployment logs). - Example: Flagging high-change-failure-rate services for refactoring. ### Technical Specifications/Examples - **Code Health Dashboard**: Tracks metrics like test coverage, cyclomatic complexity, and open bug counts. - **CI/CD Integration**: Automated alerts for degradation in DORA metrics (e.g., prolonged lead times). - **Prioritization Formula**: `Debt Score = (Impact × Urgency) / Effort` ### Key Takeaways 1. **Metrics Matter**: DORA provides actionable benchmarks for engineering efficiency. 2. **Balance Innovation and Stability**: Allocate dedicated time (e.g., 20%) for debt reduction. 3. **Culture Drives Success**: Blameless retrospectives foster accountability and continuous improvement. 4. **Tooling is Critical**: Dashboards and automation enable real-time debt visibility. ### Limitations/Caveats - **Metric Overload**: Too many KPIs can obscure focus; prioritize a core set. - **Context Sensitivity**: DORA benchmarks may not apply uniformly to all teams (e.g., legacy systems). - **Long-Term Commitment**: Debt reduction requires sustained investment beyond one-off fixes.
Messaging Patterns Explained: Pub-Sub, Queues, and Event Streams
The article explores common messaging patterns in distributed systems, focusing on Pub/Sub (Publish-Subscribe) as a scalable solution for decoupled communication. It contrasts Pub/Sub with other patterns like Point-to-Point and Request-Reply, highlighting its advantages in handling high-volume, real-time data streams. Key considerations include message brokers, topic-based routing, and trade-offs between latency and reliability. --- ### Core Technical Concepts/Technologies - **Pub/Sub (Publish-Subscribe)** - **Point-to-Point Messaging** - **Request-Reply Pattern** - **Message Brokers (e.g., Kafka, RabbitMQ)** - **Topics/Queues** - **Event-Driven Architecture** --- ### Main Points - **Pub/Sub Basics**: - Publishers send messages to topics; subscribers receive messages based on subscribed topics. - Decouples producers and consumers, enabling scalability. - **Comparison with Other Patterns**: - **Point-to-Point**: Direct communication between sender/receiver (e.g., task queues). - **Request-Reply**: Synchronous; used for immediate responses (e.g., HTTP). - **Implementation**: - Brokers (e.g., Kafka) manage topic partitioning, replication, and delivery guarantees. - Example: Kafka uses topics with partitions for parallel processing. - **Trade-offs**: - **Pros**: Scalability, loose coupling, real-time processing. - **Cons**: Complexity in message ordering, potential latency. --- ### Technical Specifications/Code Examples - **Kafka Topic Creation**: ```sh kafka-topics --create --topic orders --partitions 3 --replication-factor 2 ``` - **RabbitMQ Exchange Binding**: ```python channel.exchange_declare(exchange='logs', exchange_type='fanout') ``` --- ### Key Takeaways 1. **Scalability**: Pub/Sub handles high-volume data streams efficiently. 2. **Decoupling**: Producers/consumers operate independently. 3. **Broker Choice**: Kafka excels in throughput; RabbitMQ offers simpler setup. 4. **Latency vs. Reliability**: At-least-once delivery may increase latency. --- ### Limitations/Caveats - **Message Ordering**: Challenging in distributed brokers without partitioning. - **Complexity**: Requires tuning (e.g., partition counts, retention policies). - **Further Exploration**: Compare with streaming frameworks (e.g., Apache Pulsar).
How Halo on Xbox Scaled to 10+ Million Players using the Saga Pattern
The article explores how *Halo* on Xbox scaled to support 10 million concurrent players by leveraging distributed systems, microservices, and cloud infrastructure. Key strategies included partitioning game servers, optimizing matchmaking, and implementing robust load balancing. The technical architecture prioritized low latency, fault tolerance, and horizontal scalability. ### Core Technical Concepts/Technologies - Distributed systems - Microservices architecture - Load balancing (e.g., round-robin, least connections) - Partitioning (sharding) - Matchmaking algorithms - Cloud infrastructure (Azure) - Fault tolerance and redundancy ### Main Points - **Scalability Challenges**: Handling 10M concurrent players required overcoming network bottlenecks, server overload, and matchmaking delays. - **Server Partitioning**: Game servers were sharded geographically to reduce latency and distribute load. - **Dynamic Matchmaking**: Used algorithms to group players by skill and proximity while minimizing wait times. - **Load Balancing**: Combined round-robin and least-connections methods to evenly distribute traffic. - **Cloud Infrastructure**: Leveraged Azure for elastic scaling, allowing rapid provisioning of resources during peak times. - **Fault Tolerance**: Redundant servers and automatic failover ensured uptime during outages. ### Technical Specifications/Implementation - **Matchmaking Logic**: Prioritized latency (<50ms) and skill-based fairness (TrueSkill algorithm). - **Server Allocation**: Used Kubernetes for orchestration, dynamically scaling server instances. - **Monitoring**: Real-time metrics (e.g., player count, server health) via Prometheus/Grafana. ### Key Takeaways 1. **Partitioning is critical**: Geographic sharding reduces latency and balances load. 2. **Elastic cloud scaling**: On-demand resource allocation handles traffic spikes effectively. 3. **Optimize matchmaking**: Combine skill and latency metrics for better player experience. 4. **Redundancy ensures reliability**: Automated failover prevents downtime during failures. ### Limitations/Further Exploration - **Cost**: Cloud scaling can become expensive at extreme scales. - **Complexity**: Microservices introduce operational overhead (e.g., debugging). - **Future Work**: AI-driven matchmaking or edge computing could further optimize performance.
How Canva Collects 25 Billion Events a Day
Canva's event collection system processes 25 billion events daily, leveraging a scalable architecture with Kafka, Flink, and S3. The system prioritizes reliability, low latency, and cost-efficiency while handling diverse event types from global users. Key optimizations include batching, compression, and intelligent routing to balance performance and resource usage. --- ### Core Technical Concepts/Technologies - **Event Streaming**: Kafka for high-throughput data ingestion - **Stream Processing**: Flink for real-time event aggregation/enrichment - **Storage**: S3 for cost-effective long-term retention - **Batching/Compression**: Protocol Buffers (Protobuf) and Snappy for efficiency - **Load Balancing**: Regional routing to minimize latency --- ### Main Points - **Scale Challenges**: - 25B events/day (~300k events/sec peak) with sub-second latency requirements - Events vary in size (1KB–10KB) and type (e.g., clicks, edits, collaborations) - **Architecture**: 1. **Client SDKs**: Lightweight collectors batch events (5s/100KB thresholds) with Protobuf+Snappy compression. 2. **Ingestion Layer**: Regional Kafka clusters handle traffic spikes; auto-scaling via Kubernetes. 3. **Processing**: Flink jobs enrich/aggregate events (e.g., sessionization) in real time. 4. **Storage**: Processed data lands in S3 (Parquet format) via hourly partitions for analytics. - **Optimizations**: - **Batching**: Reduces network overhead (e.g., 100KB batches cut TCP handshake costs). - **Regional Proximity**: Clients route to nearest AWS region (us-east-1, ap-southeast-2, etc.). - **Dead-Letter Queues**: Handle malformed events without blocking pipelines. --- ### Technical Specifications - **Kafka Configuration**: - 6-node clusters per region, 32 vCPUs/node, 64GB RAM - Retention: 7 days (hot storage), 30 days (cold via S3) - **Flink Jobs**: - Checkpointing every 10s for fault tolerance - Parallelism tuned per event type (e.g., 32–128 tasks) --- ### Key Takeaways 1. **Batching is critical** for high-volume event systems to reduce network/processing overhead. 2. **Regional routing** improves latency and reliability for global user bases. 3. **Protocol Buffers + Snappy** offer an optimal balance of size and speed for serialization. 4. **Separation of hot/cold storage** (Kafka → S3) balances cost and accessibility. --- ### Limitations & Future Work - **Cold Start Latency**: Flink recovery from checkpoints can delay processing after failures. - **Schema Evolution**: Protobuf requires careful versioning for backward compatibility. - **Exploration Areas**: Testing Arrow format for analytics queries on S3 data.
EP161: A Cheatsheet on REST API Design Best Practices
This cheatsheet provides a concise guide to REST API design principles, covering best practices for endpoints, HTTP methods, status codes, versioning, authentication, and error handling. It emphasizes simplicity, consistency, and scalability while addressing common pitfalls in API development. --- ### Core Technical Concepts/Technologies - REST (Representational State Transfer) - HTTP methods (GET, POST, PUT, DELETE, PATCH) - API versioning (URL, headers) - Authentication (JWT, OAuth, API keys) - Error handling (HTTP status codes, custom error messages) - Pagination, filtering, sorting --- ### Main Points - **Endpoint Design**: - Use nouns (e.g., `/users`) instead of verbs. - Keep URLs hierarchical (e.g., `/users/{id}/posts`). - Use lowercase and hyphens for readability. - **HTTP Methods**: - `GET` for retrieval, `POST` for creation, `PUT/PATCH` for updates, `DELETE` for removal. - `PUT` replaces entire resources; `PATCH` updates partial fields. - **Status Codes**: - `2xx` for success, `4xx` for client errors, `5xx` for server errors. - Common codes: `200` (OK), `201` (Created), `400` (Bad Request), `401` (Unauthorized), `404` (Not Found). - **Versioning**: - URL-based (e.g., `/v1/users`) or header-based (`Accept: application/vnd.api.v1+json`). - Avoid breaking changes; deprecate old versions gracefully. - **Authentication**: - Prefer OAuth2 or JWT for security. - API keys for simpler use cases (rate-limited). - **Error Handling**: - Return structured errors with codes, messages, and details. - Example: ```json { "error": { "code": 404, "message": "User not found" } } ``` - **Pagination/Filtering**: - Use `limit`, `offset`, or cursor-based pagination. - Filter via query params (e.g., `/users?role=admin`). --- ### Key Takeaways 1. **Consistency**: Follow REST conventions (nouns, HTTP methods) for predictable APIs. 2. **Security**: Use standardized authentication (OAuth2/JWT) and avoid sensitive data in URLs. 3. **Clarity**: Provide meaningful status codes and error messages for debugging. 4. **Scalability**: Implement pagination and versioning early to handle growth. 5. **Maintainability**: Document APIs thoroughly and deprecate versions systematically. --- ### Limitations/Caveats - REST may not suit real-time applications (consider WebSockets/gRPC). - Over-fetching/under-fetching can occur; GraphQL is an alternative. - Versioning requires careful planning to avoid fragmentation.
How DNS Works 🔥
**1. Executive Summary** DNS (Domain Name System) servers translate human-readable domain names into machine-readable IP addresses, enabling internet communication. They operate through a hierarchical, distributed system involving root, TLD, and authoritative servers, with caching to improve efficiency. DNS queries follow a recursive or iterative resolution process, and various record types (A, CNAME, MX, etc.) serve specific functions. **2. Core Technical Concepts/Technologies** - DNS (Domain Name System) - IP address resolution - DNS hierarchy (root, TLD, authoritative servers) - Recursive vs. iterative queries - DNS record types (A, CNAME, MX, TXT, NS) - Caching and TTL (Time to Live) **3. Main Points** - **DNS Purpose**: Maps domain names (e.g., `google.com`) to IP addresses (e.g., `142.250.190.46`). - **Hierarchy**: - **Root servers**: Direct queries to TLD servers (e.g., `.com`, `.org`). - **TLD servers**: Point to authoritative servers for specific domains. - **Authoritative servers**: Store the domain’s DNS records. - **Query Process**: - **Recursive**: Resolver fetches the answer on behalf of the client. - **Iterative**: Resolver queries servers step-by-step until resolution. - **DNS Records**: - **A**: IPv4 address. - **AAAA**: IPv6 address. - **CNAME**: Alias for another domain. - **MX**: Mail server address. - **TXT**: Text metadata (e.g., SPF records). - **Caching**: DNS resolvers cache responses to reduce latency (TTL dictates cache duration). **4. Technical Specifications/Examples** - Example DNS query flow: 1. User requests `example.com`. 2. Recursive resolver queries root → TLD (`.com`) → authoritative server. 3. Authoritative server returns the A record (`93.184.216.34`). - Sample DNS records: ```plaintext example.com. A 93.184.216.34 www.example.com. CNAME example.com. example.com. MX 10 mail.example.com. ``` **5. Key Takeaways** - DNS is critical for internet functionality, translating domains to IPs. - Uses a distributed, hierarchical system for scalability and reliability. - Caching and TTL optimize performance and reduce server load. - Different record types serve distinct purposes (e.g., MX for email). - Recursive resolvers simplify queries for end users. **6. Limitations/Caveats** - DNS caching can delay updates (propagation depends on TTL). - Vulnerable to attacks like DNS spoofing (DNSSEC mitigates this). - Complex setups (e.g., load balancing) may require advanced record configurations.
Synchronous vs Asynchronous Communication: When to Use What?
### Core Technical Concepts/Technologies Discussed - Synchronous communication - Asynchronous communication - Message queues (e.g., Kafka, RabbitMQ) - Request-response vs. event-driven architectures - Latency, throughput, and scalability considerations ### Main Points - **Synchronous Communication**: - Real-time, blocking interaction (e.g., HTTP/RPC). - Pros: Simplicity, immediate feedback. - Cons: Tight coupling, scalability challenges due to waiting. - **Asynchronous Communication**: - Non-blocking, decoupled (e.g., message queues, event streaming). - Pros: Scalability, fault tolerance, better resource utilization. - Cons: Complexity in error handling and eventual consistency. - **Use Cases**: - Synchronous: Low-latency needs (e.g., user authentication). - Asynchronous: High-throughput tasks (e.g., order processing, logs). - **Technical Specs/Examples**: - Synchronous: REST APIs, gRPC. - Asynchronous: Kafka (persistent logs), RabbitMQ (message brokering). ### Key Takeaways 1. **Trade-offs**: Synchronous for simplicity; asynchronous for scalability. 2. **Decoupling**: Asynchronous systems reduce dependencies but require robust error handling. 3. **Tool Choice**: Kafka excels in high-volume event streaming; RabbitMQ for flexible messaging. ### Limitations/Further Exploration - Synchronous: Struggles under high load; retries can compound latency. - Asynchronous: Debugging and monitoring are harder in distributed systems. - Hybrid approaches (e.g., async APIs with sync wrappers) warrant deeper analysis.
How Meta Built Threads to Support 100 Million Signups in 5 Days
Meta built Threads to handle massive scale by leveraging Instagram's infrastructure while optimizing for rapid development. The system prioritizes high availability, low latency, and efficient scaling using a combination of microservices, caching, and distributed databases. Key innovations include read-after-write consistency, multi-region replication, and a hybrid approach to data partitioning. ### Core Technical Concepts/Technologies - Microservices architecture - Distributed databases (e.g., Cassandra, TAO) - Caching (Memcached, TAO) - Read-after-write consistency - Multi-region replication - Data partitioning (hybrid approach) - Rate limiting and load shedding ### Main Points - **Leveraged Instagram's Infrastructure**: Threads reused Instagram's authentication, graph data, and existing microservices to accelerate development. - **Scalable Data Storage**: - Used Cassandra for scalable, distributed storage with eventual consistency. - Implemented TAO (a graph database) for low-latency reads and writes. - **Consistency Model**: - Ensured read-after-write consistency for user posts by routing reads to the primary region temporarily. - **Multi-Region Deployment**: - Deployed across multiple AWS regions for fault tolerance and reduced latency. - Used asynchronous replication for cross-region data sync. - **Performance Optimizations**: - Heavy use of caching (Memcached) to reduce database load. - Implemented rate limiting and load shedding to handle traffic spikes. - **Data Partitioning**: - Hybrid approach: some data (e.g., posts) sharded by user ID, while other data (e.g., timelines) used a fan-out model. ### Technical Specifications/Implementation Details - **Cassandra**: Used for scalable storage with tunable consistency levels. - **TAO**: Optimized for low-latency access to graph data (e.g., follower relationships). - **Memcached**: Cache layer to reduce read latency and database load. - **Rate Limiting**: Implemented at the API gateway layer to prevent abuse. ### Key Takeaways 1. **Reuse Existing Infrastructure**: Leveraging Instagram's systems allowed Threads to launch quickly at scale. 2. **Prioritize Consistency Where Needed**: Read-after-write consistency was critical for user experience. 3. **Design for Multi-Region Resilience**: Asynchronous replication and regional failover ensured high availability. 4. **Optimize for Read Heavy Workloads**: Caching and efficient data partitioning reduced latency. 5. **Plan for Traffic Spikes**: Rate limiting and load shedding prevented outages during peak loads. ### Limitations/Caveats - Eventual consistency in Cassandra can lead to temporary data discrepancies. - Multi-region replication adds complexity to data synchronization. - The hybrid partitioning approach requires careful tuning to balance load. - Further optimizations may be needed as user growth continues.
How WhatsApp Handles 40 Billion Messages Per Day
WhatsApp efficiently handles 40 billion daily messages through a distributed architecture leveraging Erlang/OTP for concurrency, end-to-end encryption via the Signal Protocol, and optimized data routing. Key components include a load-balanced server fleet, message queuing with in-memory storage, and horizontal scaling to manage peak loads while maintaining low latency and high reliability. ### Core Technical Concepts/Technologies - **Erlang/OTP**: For high concurrency and fault tolerance - **Signal Protocol**: End-to-end encryption (E2EE) - **Distributed Systems**: Load balancing, sharding, and horizontal scaling - **In-Memory Storage**: Ephemeral message queuing (RAM) - **XMPP (modified)**: Lightweight messaging protocol ### Main Points - **Architecture**: - **Stateless Servers**: Handle authentication/encryption; scale horizontally. - **Message Queues**: Stored in RAM for low-latency delivery; persisted only if offline. - **Load Balancing**: Distributes traffic evenly across global data centers. - **Encryption**: - E2EE implemented via Signal Protocol, with keys exchanged during session setup. - Metadata minimized to enhance privacy. - **Optimizations**: - **Message Batching**: Reduces overhead by grouping acknowledgments. - **Connection Pooling**: Reuses TCP connections to minimize latency. - **Sharding**: User data partitioned by unique ID for parallel processing. - **Scalability**: - **Read Replicas**: Handle read-heavy workloads (e.g., group chats). - **Automatic Failover**: Erlang’s "let it crash" philosophy ensures resilience. ### Technical Specifications - **Protocol**: Modified XMPP (reduced overhead vs. HTTP). - **Storage**: Messages deleted from servers after delivery; offline messages use SQLite. - **Code Example**: Erlang’s gen_server behavior manages message queues (not shown in detail). ### Key Takeaways 1. **Concurrency First**: Erlang/OTP enables handling millions of simultaneous connections. 2. **Ephemeral Storage**: RAM-based queues prioritize speed, with persistence as fallback. 3. **Privacy by Design**: E2EE and minimal metadata collection are core tenets. 4. **Horizontal Scaling**: Stateless services and sharding support massive growth. 5. **Protocol Efficiency**: Custom XMPP reduces bandwidth vs. traditional HTTP. ### Limitations/Caveats - **Metadata Exposure**: While messages are encrypted, sender/receiver and timestamps are visible. - **Offline Storage**: SQLite may bottleneck under extreme load. - **Global Consistency**: Trade-offs exist in multi-region replication (e.g., eventual consistency). *Areas for Exploration*: - Quantum-resistant encryption upgrades. - Edge computing for further latency reduction.
Top 10 awesome MCP servers that can make your life easier 🪄✨
As AI agents become increasingly central to real-world workflows, seamless integration with external systems is no longer optional — it's essential. Model Context Protocol (MCP) servers are emerging as critical infrastructure, enabling AI to connect with platforms like Notion, Figma, Supabase, and Firecrawl. This evolution mirrors broader industry trends toward modular, API-driven AI architectures where agents not only reason but act autonomously. The rise of MCP servers signals a shift from isolated AI models to **ecosystem-aware AI systems**. Tools like Supabase MCP for database operations and ElevenLabs MCP for synthetic voice generation showcase how AI can perform high-value tasks with minimal friction. Organizations investing early in agentic platforms and MCP integrations are likely to see significant efficiency and innovation gains. Here’s a clean summary of the 10 MCP servers mentioned in the article: 1. **Notion MCP** → Interact with Notion workspaces: create pages, databases, and update content via AI. 2. **Figma MCP** → Access and modify Figma designs: search files, get frames, and even generate designs. 3. **Supabase MCP** → Manage Supabase projects: create tables, run SQL queries, and interact with database rows. 4. **Firecrawl MCP** → Crawl websites and extract structured data easily — perfect for agents needing fresh content. 5. **Browserless MCP** → Control headless browsers: take screenshots, run scraping tasks, and test web apps. 6. **Docs GPT MCP** → Help agents deeply understand documentation by fetching content from technical docs. 7. **Dynamo MCP** → Perform structured actions like filling forms, running tasks, and updating records. 8. **ElevenLabs MCP** → Generate synthetic voice content (text-to-speech) for use cases like audiobooks or UIs. 9. **Discord MCP** → Interact with Discord servers: send messages, manage channels, and automate bots. 10. **AssemblyAI MCP** → Access transcription, summarization, and audio intelligence features for speech data. Each MCP server allows AI agents to **do real-world tasks** by plugging into different tools and services — supercharging app capabilities.
EP160: Top 20 System Design Concepts You Should Know
This article outlines 20 essential system design concepts crucial for building scalable, reliable, and efficient distributed systems. It covers foundational principles like load balancing, caching, and databases, as well as advanced topics such as consensus algorithms and microservices. The guide serves as a comprehensive reference for engineers preparing for system design interviews or real-world implementations. ### Core Technical Concepts/Technologies Discussed - Load Balancing - Caching (CDN, Redis, Memcached) - Databases (SQL, NoSQL, Sharding, Replication) - Proxies (Forward/Reverse) - CAP Theorem - ACID vs. BASE - Consistent Hashing - Leader Election - Message Queues (Kafka, RabbitMQ) - Microservices - Rate Limiting - Distributed File Systems (HDFS) - Peer-to-Peer Networks - Polling vs. Webhooks - Heartbeat Mechanism - Checksum - API Gateway - SLA, SLO, SLI - Redundancy & Replication - Consensus Algorithms (Paxos, Raft) ### Main Points - **Load Balancing**: Distributes traffic across servers to optimize resource use (e.g., Round Robin, Least Connections). - **Caching**: Reduces latency by storing frequently accessed data (CDNs for static content, Redis/Memcached for dynamic data). - **Database Scaling**: Vertical (upgrading hardware) vs. horizontal (sharding) scaling; replication ensures high availability. - **CAP Theorem**: Trade-offs between Consistency, Availability, and Partition Tolerance in distributed systems. - **Microservices**: Decouples functionality into independent services, improving scalability but adding complexity. - **Rate Limiting**: Protects systems from abuse (e.g., token bucket, leaky bucket algorithms). - **Consensus Algorithms**: Paxos/Raft ensure agreement in distributed systems despite failures. ### Technical Specifications & Examples - **Consistent Hashing**: Minimizes data redistribution when nodes are added/removed (used in DynamoDB, Cassandra). - **Leader Election**: ZooKeeper’s Zab protocol or Raft for coordinating distributed systems. - **Message Queues**: Kafka’s pub-sub model vs. RabbitMQ’s queue-based messaging. ### Key Takeaways 1. **Trade-offs are fundamental**: CAP theorem and ACID vs. BASE highlight the need to prioritize based on use cases. 2. **Scalability requires planning**: Techniques like sharding, caching, and load balancing are critical for growth. 3. **Redundancy ensures reliability**: Replication and heartbeat mechanisms prevent single points of failure. 4. **Microservices offer flexibility**: But require robust API gateways and monitoring (SLOs/SLIs). 5. **Real-world systems combine multiple concepts**: E.g., Netflix uses CDNs, microservices, and rate limiting. ### Limitations & Further Exploration - Some concepts (e.g., Paxos) are complex to implement and may require deeper study. - Emerging technologies (e.g., serverless, service mesh) could expand this list. - Practical implementation details (e.g., tuning Redis eviction policies) are beyond the scope.